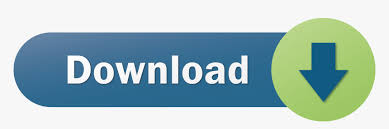
MaxBulk Mailer 5.72 serial key or number

MaxBulk Mailer 5.72 serial key or number
Elucidating the Crucial Role of Hole Injection Layer in Degradation of Organic Light-Emitting Diodes
Terms & Conditions
Electronic Supporting Information files are available without a subscription to ACS Web Editions. The American Chemical Society holds a copyright ownership interest in any copyrightable Supporting Information. Files available from the ACS website may be downloaded for personal use only. Users are not otherwise permitted to reproduce, republish, redistribute, or sell any Supporting Information from the ACS website, either in whole or in part, in either machine-readable form or any other form without permission from the American Chemical Society. For permission to reproduce, republish and redistribute this material, requesters must process their own requests via the RightsLink permission system. Information about how to use the RightsLink permission system can be found at http://pubs.acs.org/page/copyright/permissions.html.
[Studies in Classification Data Analysis and Knowledge Organization] Vladimir Batagelj Hans-Hermann Bock Anuka Ferligoj Ale iberna - Data Science and Classification (2006 S.pdf
burndy catalogo .pdf
Experience. Technology. Answers.
TM
BURNDY offers a variety of products and
®
solutions to serve the following industries: data
centers, OEM, oil and gas, renewable energy,
power generation and telecommunications
Table of Contents
Products
Mechanical......................................................... 1-31
Oxide Inhibitor...................................................26-27
Grounding.........................................................32-64
Cable Ties......................................................... 65-70
Small Compression........................................... 71-90
Medium and Large Compression......................91-151
Tooling...........................................................152-189
Index................................................................... 190
New Items
BBB Copper BusBar..........................................43-45
TM
BDT BONDIT ...................................................28-29
TM
Blank Tongue HYLUG
Copper Code Terminals...............................131-133
TM TM
BREAK-AWAY HYLUG
Copper and Aluminum Compression
Terminals...................................................127-130
® TM
BURNDYWeld QIKLITE
Exothermic Battery Ignition System.................... 57
Ferrules
Bare and Insulated........................................ 71-81
TM
HYLUG
Narrow Tongue Copper Compression
Terminals........................ 94-95, 102-103, 108-109
®
PATRIOT PAT750-LI
Lithium-Ion Battery Actuated Tool......................174
Pool Water Bonding Kit.......................................... 30
®
The SmartCart
Modular Wire Management System........... 186-189
TM
U-BLOK
Power Distribution System...................................12
U-Die Kits
®
Copper, Aluminum and HYGROUND ..................157
Reconnect to www.burndy.com!
BURNDY® EC&M Mechanical
TYPES KS & KS-3
SERVIT® Split Bolts
For Copper, Copperweld
Compact, high strength, high copper alloy SERVIT® split-bolt has free-
running threads and easy to grip wrench flats. Highly resistant to season
cracking and corrosion, the SERVIT® provides maximum pressure and as-
sures a secure connection on all combinations of run and tap conductors.
Type KS-3 accommodates 3 maximum size conductors.
Copper Recommended ▲
Catalog Range for Equal Min. Tap with Tightening
Number Run and Tap Max. Run Torque in-lb
KS90 12 - 10 str. 16 str.
80
KS15** 10 - 8 str. 14 str.
KS17** 8 str. - 6 sol. 14 str.
KS17-3* 8 str. - 6 sol. 16 str.
165
KS20** 8 str. - 4 sol. 14 str.
KS20-3* 8 str. - 4 sol. 14 str.
KS22** 6 str. - 2 sol. 14 str.
KS22-3* 6 str. - 2 sol. 14 str. 275
KS23** 6 - 2 str. 14 str.
KS25** 4 - 1/0 str. 14 str.
385
KS26** 2 - 2/0 str. 14 str.
KS27** 1 - 3/0 str. 8 sol. 500
KS29** 1 str. - 250 kcmil 8 str.
650
KS31** 1/0 str. - 350 kcmil 1/0 str.
KS34** 2/0 str. - 500 kcmil 2/0 str. 825
KS39 4/0 str. - 750 kcmil 4/0 str. 1000
KS44 300 - 1000 kcmil 4/0 str. 1100
* Not UL Listed or CSA Certified. Consult UL486 tables 7-4, 7-5, 7-6 for smaller
** UL Rated for Direct Burial conductor combinations.
▲ Listed torque values are for maximum
conductor combinations accommodated.
TYPE KSU
Universal SERVIT®
486A
Copper only
Tin plated service connector with spacer bar for all combinations of copper,
aluminum, ACSR, 6201, 5005, and steel.
Run Tap Recommended
Catalog Copper & Run ACSR Copper & Tap ACSR* Tightening
Number Aluminum AAAC, 5005 Aluminum AAC, 5005 Torque in-lb
KSU17 12 - 6 sol. 8 (6-1) 12 sol. - 6 sol. 8 (6-1)
165
KSU20 10 - 4 sol. 6 (6-1) 10 sol. - 4 sol. 6 (6-1)
6 (6-1) - 6 (6-1) -
KSU22 10 - 2 sol. 10 sol. - 2 sol.
4 (7-1) 4 (7-1)
275
3 (6-1) - 6 (6-1) -
KSU23 8 - 2 str. 8 sol. - 2 str.
2 (6-1) 2 (6-1)
3 (6-1) - 6 (6-1) -
KSU25 2 - 1/0 str. 10 str. - 1/0 str.
1 (6-1) 1 (6-1)
385
2 (6-1) - 6(6-1) -
KSU26 2 - 2/0 str. 8 str. - 2/0 str.
1/0 ( 6-1) 1/0 (6-1)
1 (6-1) - 6 (6-1) -
KSU27 1 - 3/0 str. 8 sol. - 3/0 str. 500
2/0 (6-1) 2/0 (6-1)
1 str. - 2/0 (6-1) - 6( 6-1) -
KSU29 8 str. - 250
250 kcmil 4/0 (6-1) 4/0 (6-1)
650
4/0 str. - 3/0 (6-1) - 4 (6-1) -
KSU31 4 str. - 350
350 kcmil 4/0 ( 6-1) 4/0 (6-1)
400 - 336 (30-7) - 2 (6-1) -
KSU34 2 str. - 500 825
500 kcmil 477 (18-1) 477 (18-1)
* Accommodates compressed conductors within conductor ranges.
1
Mechanical BURNDY® EC&M BURNDY® EC&M Mechanical
TYPE KVS TYPE KSA
OKLIP™ TRITAP™ SERVIT® Tap Connector
486B
Mechanical Connector for Copper and Copperweld. Compact, two-piece,
An aluminum tin-plated universal tap connector for all combinations of alu-
high strength, high copper alloy BURNDY® OKLIP™ recommended for heavy
minum to aluminum, aluminum to copper and copper to copper applications.
duty connections. Neoprene rings hold DURIUM™ bolts in place during
UL486B Listed. 90° C rated.
installation. Installed with ordinary wrench.
Recommended
Catalog Copper Recommended Catalog Run Run Tap Tap Tightening
Number Run Tap Tightening Torque in-lb Number Min. Max. Min. Max. Torque in-lb ▲
KVS26 2 - 2/0 str. 6 sol. - 2/0 str. 180 KSA6 10 sol. 6 str. 10 sol. 6 str.
KVS28 1/0 - 4/0 str. 10 - 4/0 str. 250 165
KSA4 8 sol. 4 str. 10 sol. 4 str.
KVS31 250 - 350 kcmil 10 str. - 350 kcmil 325 KSA2 6 sol. 2 str. 8 str. 2 str. 275
KVS34 400 - 500 kcmil 10 str. - 500 kcmil 375 KSA1/0 2 compact 1/0 str. 8 sol. 1/0 str.
KVS40 400 - 800 kcmil 3/0 str. - 800 kcmil 385
500 KSA2/0 2 compact 2/0 str. 8 str. 2/0 str.
KVS44 500 - 1000 kcmil 3/0 str. - 1000 kcmil KSA4/0 2 compact 4/0 str. 6 str. 4/0 str. 500
KSA350* 1/0 compact 350 kcmil 4 str. 350 kcmil 650
KSA500* 400 compact 500 kcmil 2 compact 500 kcmil 825
*Not CSA Listed.
TYPE KVSU ▲ Listed torque values are for maximum conductor combinations accommodated.
Universal OKLIP™
TYPE SC
SERVIT® Cover
Mechanical Connector for all combinations of Copper, Aluminum, ACSR,
AAAC and 5005. Compact, high strength, tin plated copper alloy two-piece
connector with spacer and tin-plated silicon bronze DURIUM™ hardware.
Recommended for heavy duty connections. Spacer separates dissimilar con-
ductors and provides long contact length. Neoprene ring prevents loss of Range-taking, insulating cover for SERVIT® and equivalent split-bolt
shorter bolt during installation. Longer peened bolt permits swivel action for connectors.
easier installation.
Catalog
Run Tap Number For Use With
ACSR, ACSR, Recommended SC4 KS17, KS17-3, KS20, KSU17, KSU20
Catalog Copper & AAAC, & Copper & AAAC, & Tightening SC2 KS22, KS20-3, KS23, KS22-3, KSA6, KSA4, KSU22, KSU23
Number Aluminum 5005 Aluminum 5005 Torque in-lb SC2/0 KS25, KS26, KSA2, KSA 1/0, KSU25, KSU26
KVSU26 2 - 2/0 str. 3 - 2/0 6 - 2/0 str. 6 - 2/0 180
KVSU28 1/0 - 4/0 str. 1/0 - 4/0 6 - 4/0 str. 6 - 4/0 250
250 - 350 6 str. - 350
KVSU31 4/0 - 300 6 - 300 325
kcmil kcmil
KVSU34
400 - 500 336.4- 4 str. - 500
5 - 397.5 375
TYPE QPX
kcmil 397.5 kcmil VERSITAP™ Parallel Clamp
400 - 800 4/0 str. - 800
KVSU40 4/0 - 800 3/0 - 715.5
kcmil kcmil
500
500 -1000 4/0 str. -1000
KVSU44 4/0 -1000 4/0 - 900
kcmil kcmil
Parallel clamp for wide range of copper cable. Makes parallel tap, tees,
crosses and end-to-end connections. Rounded edges for easy taping.
Cable Range Recommended
Catalog Run Run Tap Tap Tightening
Number Min. Max. Min. Max. Torque in-lb
QPX2C2C 6 str. 2 str. 6 str. 2 str. 150
QPX282C 1 str. 4/0 str. 6 str. 2 str.
250
QPX2828 1 str. 4/0 str. 1 str. 4/0 str.
QPX342C 250 kcmil 500 kcmil 6 str. 2 str.
QPX3428 250 kcmil 500 kcmil 1 str. 4/0 str. 375
QPX3434 250 kcmil 500 kcmil 250 kcmil 500 kcmil
QPX442C 500 kcmil 1000 kcmil 6 str. 2 str.
QPX4428 500 kcmil 1000 kcmil 1 str. 4/0 str.
500
QPX4434 500 kcmil 1000 kcmil 250 kcmil 500 kcmil
QPX4444 500 kcmil 1000 kcmil 500 kcmil 1000 kcmil
2 3
Mechanical BURNDY® EC&M BURNDY® EC&M Mechanical
TYPE QPX-Y TYPE KA
Universal VERSITAP™ KA-LUG™ Terminal
Parallel Clamp For Copper Cable
Universal parallel clamp for copper and aluminum. Tin-plated. Makes paral-
lel taps, tees, crosses or end-to-end connections. Compact, economical, high copper alloy terminal for joining a wide range of
Conductor Range cable to equipment pads or terminal blocks.
Catalog Aluminum or Copper Catalog Conductor
Number Run Tap Number Range
QPX2C2C-Y 6 - 2 str. 6 - 2 str. KA8C 14 sol. - 8 str.
QPX282C-Y 1 - 4/0 str. 6 - 2 str. KA4C 14sol. - 4 str.
QPX2828-Y 1/0 - 4/0 str. 1/0 - 4/0 str. KA25 4 - 1/0 str.
QPX342C-Y 250 - 500 kcmil 6 - 2 str. KA25-2TC38 4 - 1/0 str.
QPX3428-Y 250 - 500 kcmil 1/0 - 4/0 str. KA28 1 - 4/0 str.
QPX3434-Y 250 - 500 kcmil 250 - 500 kcmil KA34 4/0 str. - 500 kcmil
QPX4444-Y 750 - 1000 kcmil 750 - 1000 kcmil
TYPE QA
TYPES KPA, KPA-UP QIKLUG™ Terminal
SCRULUG™ Terminal
For Copper Cable
Heavy-duty terminal for a wide range of copper cables. One-hole tongue.
Figure 2
Figure 1 Catalog Conductor Stud
High copper alloy terminal for joining a wide range of cable to equipment Number Range Size
pads or terminal blocks. Plain copper finish. QA8C-B 14 sol. - 8 str. 10
Catalog Catalog QA4C-B 8 - 4 str. 1/4
Number Number Conductor QA1C-B 4 - 1 str. 1/4
Fig. 1 Fig. 2 Range QA26-B 1/0 - 2/0 str. 3/8
QA28-B 3/0 - 4/0 str. 3/8
KPA8C KPA8CUP* 14 sol. - 6 str.
QA31-B 250 - 350 kcmil 1/2
KPA4C KPA4CUP* 14 sol. - 4 str.
QA34-B 400 - 500 kcmil 1/2
KPA25 — 6 - 1/0 str.
QA40-B 600 - 800 kcmil 5/8
KPA28 — 6 - 4/0 str.
QA44-B 850 - 1000 kcmil 5/8
KPA34 — 2/0 str. - 500 kcmil
QA46-B 1100 - 1500 kcmil 3/4
* For tin plating drop “UP” suffix and add “TP” suffix.
TYPES QA-2, QA-4
TYPE KLU QIKLUG™ Terminal
SCRULUG™ Terminal
For Copper Cable
Offset Tongue Non-plated
Heavy-duty terminal for a wide range of copper cables. Two-hole and four-
hole tongues.
Catalog Conductor Stud Stud
High copper alloy terminal with offset tongue for joining a wide range of cable Number Range Hole Size Hole Centers
to equipment pads or bar. Easy to install with screwdriver or wrench. Con- QA8C-2B 14 sol. - 8 str. 10 5/8
nector is reusable. Plain copper finish. QA4C-2B 8 - 4 str. 1/4 5/8
QA1C-2B 4 - 1 str. 5/16 7/8
Catalog Conductor
QA26-2B 1/0 - 2/0 str. 3/8 1
Number Range QA28-2B 3/0 - 4/0 str. 3/8 1
KLU25 14 - 10 sol. QA31-2B 250 - 350 kcmil 3/8 1
KLU35 14 sol. - 6 str. QA26-2N 1/0 - 2/0 str. 1/2 1-3/4
KLU70 8 sol. - 2 str. QA28-2N 3/0 - 4/0 str. 1/2 1 -3/4
KLU125 2 - 1/0 str. QA31-2N 250 - 350 kcmil 1/2 1-3/4
KLU175 4 - 3/0 str. QA34-2B 400 - 500 kcmil 3/8 1
KLU225 2 - 4/0 str. QA34-2N 400 - 500 kcmil 1/2 1-3/4
KLU300 1/0 str. - 350 kcmil QA34-4B 400 - 500 kcmil 3/8 1
KLU400 1/0 str. - 500 kcmil QA40-2N 600 - 800 kcmil
QA44-2N 850 - 1000 kcmil
1/2 1-3/4
QA44-4N 850 - 1000 kcmil
QA46-2N 1100 - 1500 kcmil
4 5
Mechanical BURNDY® EC&M BURNDY® EC&M Mechanical
TYPE QDA TYPE KA-U
QIKLUG™ Terminal Universal Terminal
(1 Conductor)
For Aluminum and Copper Conductors
Fig. 1
Heavy-duty terminal for a wide range of cable to equipment studs. One-hole Fig. 2
tongue finished on both sides.
Catalog Conductor Stud These dual-rated one conductor lugs are constructed from high strength alu-
Number Range Size minum alloy and electro tin-plated to provide low contact resistance.
QDA8C 14 sol. - 8 str. 3/8 Catalog Figure Recommended Tightening
QDA4C 8 - 4 str. 3/8 Number Conductor Range Number Torque (in-lb) ▲
QDA1C 4 - 1 str. 3/8
KA6U 14 - 6 str. 1 45
QDA26 1/0 - 2/0 str. 1/2
KA2U 14 - 2 str. 1 50
QDA28 3/0 - 4/0 str. 1/2
KA25U 14 - 1/0 str. 1 50
QDA31 250 - 500 kcmil 5/8
KA26U 6 - 2/0 str. 1 120
QDA34 400 - 500 kcmil 3/4
KA29U 6 str. - 250 kcmil 2 275
QDA40 600 - 800 kcmil 1
KA30U 6 str. - 300 kcmil 2 275
KA31U 6 str. - 350 kcmil 2 275
KA34U 4 str. - 500 kcmil 2 500
KA36U 2 str. - 600 kcmil 2 500
TYPES Q2A-2, Q2A-2N, Q2A-4N KA40U 300 - 800 kcmil 2 550
QIKLUG™ Terminal KA44U 500 - 1000 kcmil 2 550
▲ Listed torque values are for maximum conductor size accommodated.
Heavy-duty terminal for a wide range of copper cable. Joins two cables to
equipment pads or bars. Two hole and four hole tongue.
TYPE K2A-U
Catalog Conductor Stud Stud
Number Range Hole Size Hole Centers Universal Terminal
Q2A1C-2 4 - 1 str. 3/8 1 (2 Conductors) Fig. 1
Q2A26-2N 1/0 - 2/0 str. For Aluminum and Copper Conductors
Q2A28-2N 3/0 - 4/0 str.
Q2A31-2N 250 - 350 kcmil
Q2A34-2N 400 - 500 kcmil Fig. 2
Q2A40-2N 600 - 800 kcmil Fig. 3
Q2A28-4N 3/0 - 4/0 str. 1/2 1-3/4 Compact, wide-range, tin-plated aluminum terminal for use with two copper
Q2A31-4N 250 - 350 kcmil or aluminum cables.
Q2A34-4N 400 - 500 kcmil
Stud Stud Recommended
Q2A40-4N 600 - 800 kcmil
Q2A44-4N 850 - 1000 kcmil
Catalog Conductor Hole Hole Fig. Tightening
Q2A46-4N 1100 - 1500 kcmil Number Range Size Spacing No. Torque (in-lb) ▲
K2A25U Two: 14 - 1/0 str. 1/4 — 1 50
K2A26U Two: 14 - 2/0 str. 1/4 — 1 120
K2A29U Two: 6 str. - 250 kcmil 3/8 — 2 275
TYPES Q3A-2N, Q3A-4N K2A31U Two: 4 str. - 350 kcmil 1/2 — 2 275
K2A36U Two: 2 str. - 600 kcmil 1/2 — 2 375
QIKLUG™ Terminal
K2A40U Two: 300 - 800 kcmil 5/8 — 2 550
K2A44U Two: 500 - 1000 kcmil 5/8 — 2 550
K2A31U-2N* Two: 6 str. - 350 kcmil 1/2 1-3/4 3 275
K2A36U-2N* Two: 2 str. - 600 kcmil 1/2 1-3/4 3 375
K2A40U-2N* Two: 300 - 800 kcmil 1/2 1-3/4 3 375
Heavy-duty terminal for a wide range of copper cable. Joins three cables to K2A44U-2N* Two: 500 - 1000 kcmil 1/2 1-3/4 3 375
equipment pads or bars.
* Tongue holes drilled per NEMA standards.
Catalog Conductor Stud Stud ▲ Listed torque values are for maximum conductor size accommodated.
Number Range Hole Size Hole Centers
Q3A28-2N 3/0 - 4/0 str.
Q3A31-2N 250 - 350 kcmil
Q3A34-2N 400 - 500 kcmil
Q3A28-4N 3/0 - 4/0 str.
Q3A31-4N 250 - 350 kcmil
1/2 1-3/4
Q3A34-4N 400 - 500 kcmil
Q3A40-4N 600 - 800 kcmil
Q3A44-4N 850 - 1000 kcmil
Q3A46-4N 1100 - 1500 kcmil
Q3A48-4N 1600 - 2000 kcmil
6 7
Mechanical BURNDY® EC&M BURNDY® EC&M Mechanical
TYPES K3A-U, KK3A-U TYPE CL50-1
Universal Terminal Copper Lay-In QIKLUG™
(3 Conductor) For Copper
For Aluminum & Copper Conductors Fig. 1
Fig. 2
The Lay-In QIKLUG™ is manufactured from high strength pure electrolytic
Dual-rated three conductor lugs are constructed from high strength aluminum copper to ensure maximum strength and conductivity. UL467 Listed for Direct
alloy and electro tin-plated to provide low contact resistance. Burial in earth or concrete. The open-faced design allows for fast lay-in of
the conductor without the need for cutting or breaking.
Catalog Conductor Stud Fig. Recommended Tight-
Number Range Hole Size No. ening Torque (in-lb) ▲ Catalog Number Conductor Range Copper Stud Hole
K3A2U-2* Three: 14 - 2 str. 5/16 1 50 CL50-1 14 - 4 AWG 10
K3A25U-2* Three: 14 - 1/0 str. 3/8 1 50 NOTE: Stainless Steel Screws.
K3A26U-2N Three: 14 - 2/0 str. 1/2 1 50
K3A27U-2N Three: 6 - 3/0 str. 1/2 1 275
K3A29U-2N Three: 6 str. - 250 kcmil 1/2 1 275
K3A31U-2N Three: 6 str. - 350 kcmil 1/2 1 275
K3A36U-2N Three: 2 str. - 600 kcmil 1/2 1 375
KK3A36U-2N Three: 2 str. - 600 kcmil 1/2 2 375
TYPE KPB
KK3A40U-2N Three: 300 - 800 kcmil 1/2 2 375 For Copper
KK3A44U-2N Three: 500 - 1000 kcmil 1/2 2 375
* Slotted screw.
“N” indicates NEMA Standard holes.
▲ Listed torque values are for maximum conductor size accommodated.
UL467 Listed for direct burial application in earth or concrete.
Catalog Number Cable Range Stud Hole
TYPE K4A-U KPB4CG1 10 - 4 str. 10*
* To be assembled with TMH322 stainless steel hardware kit, ordered separately.
Universal Terminal
(4 Conductor)
For Aluminum and Copper Conductors
Fig. 1
TYPE K11A-U
Fig. 2
Universal Terminal Fig. 2 Fig. 3
These dual-rated four conductor lugs are constructed from high strength For Aluminum and
aluminum alloy and electro tin-plated to provide low contact resistance. Fig. 1
Copper Conductors
Catalog Conductor Stud Fig. Recommended Tight-
Number Range Hole Size No. ening Torque (in-lb) ▲
K4A29U-4N Four: 6 str. - 250 kcmil 1/2 1 275 Fig. 4 Fig. 5
K4A31U-4N Four: 6 str. - 350 kcmil 1/2 1 275
Dual-rated panelboard lugs are constructed from high strength extruded alu-
KK4A36U-4N Four: 2 str. - 600 kcmil 1/2 2 375
minum alloy and electro tin-plated to provide low contact resistance.
KK4A40U-4N Four: 300 - 800 kcmil 1/2 2 375
“N” indicates NEMA Standard holes.
Catalog Conductor Stud Fig. Recommended Tight-
▲ Listed torque values are for maximum conductor size accommodated. Number Range Hole Size No. ening Torque (in-lb) ▲
K11A30U* Two: 6 str. - 300 kcmil 5/16 1 275
K11A34U-2 Two: 4/0 str. - 500 kcmil 1/4 2
K11A36U-2 Two: 2 str. - 600 kcmil 3/8 3
K21A36U-2 Three: 2 str. - 600 kcmil 3/8 4
TYPE BGBL K22A36U-2 Four: 2 str. - 600 kcmil 3/8 5
375
Lay-In QIKLUG™ K11A39U-2 Two: 1/0 str. - 750 kcmil 3/8 3
*UL Listed 90°C, 600 V K22A39U-2 Four: 1/0 str. - 750 kcmil 3/8 5
* Not CSA listed.
▲ Listed torque values are for maximum conductor size accommodated.
The Lay-In QIKLUG™, type BGBL is manufactured from high strength 6061-
T6 aluminum, and is ideally suited for grounding and bonding applications
accommodating both copper and aluminum conductor sizes 14 AWG to 250
kcmil.
Catalog Number Conductor Range Hex Size
BGBL-4 14 - 4 str. Slot
BGBL-1/0 14 - 1/0 str. Slot
BGBL-250 6 str. - 250 kcmil 7/32
8 9
Mechanical BURNDY® EC&M BURNDY® EC&M Mechanical
TYPE KAU-KIT TYPE AMS
Transformer Lug Kit Dual Rated Splicer/Reducer
For Copper and Aluminum Cable
Each kit contains the UL Listed and CSA certified AL/CU rated aluminum set
screw connectors and tongue mounting hardware needed to terminate alu- All splicer/reducers are dual rated for use with aluminum and copper
minum or copper cables in “dry type” transformers. The KVA rating gives an conductors and are constructed from high strength, tin-plated aluminum.
approximate cross reference to the appropriate kit. PENETROX™ oxide inhibiting joint compounds are recommended for all alu-
minum applications.
Terminals
Catalog Transformer Catalog Catalog Wire Range
Number KVA Rating Qty Number Conductor Range Number Min. Max.
AMS-2* 14 AWG 2 AWG
15-37.5 1Ø 8 KA2U 14 - 2 str.
KAU-KIT1 AMS-0* 8 AWG 1/0 AWG
15-45 3Ø 4 KA29U 6 str. - 250 kcmil
AMS-4/0 6 AWG 4/0 AWG
50-75 1Ø
KAU-KIT2 12 KA29U 6 str. - 250 kcmil AMS-250 6 AWG 250 kcmil
75-112.5 3Ø
AMS-350 6 AWG 350 kcmil
100-167 1Ø 6 K2A31U 6 str. - 350 kcmil
KAU-KIT3 AMS-500 3/0 AWG 500 kcmil
150-300 3Ø 7 K2A40U 300 - 800 kcmil
AMS-750 250 kcmil 750 kcmil
KAU-KIT4 400-500 3Ø 15 K2A40U 300 - 800 kcmil
AMS-1000 500 kcmil 1000 kcmil
* Slotted Screws
Catalog Hardware
Number Qty. Bolt Size Qty. Nut Qty. Washer
Captive
KAU-KIT1 8 1/4-20 x 3/4 HH 8 1/4 x 20 HN —
To Nut
8 1/4-20 X 3/4 HH Captive
TYPE UGSKIT
KAU-KIT2 16 1/4 x 20 HN — Watertight/Underground Splice Kit
8 1/4-20 x 2 HH To Nut
KAU-KIT3
5 1/2-13 x 2 HH
11 1/2-13 HN
22 1/2 FW For all Aluminum or
6 1/2-13 x 2-1/2 HH 11 1/2 Belleville Copper/Aluminum Combinations
7 1/-13 x 2 22 1/2 FW
KAU-KIT4 11 1/2-13 HN
4 1/2-13 x 2-1/2 11 1/2 Belleville Fig. 1 Fig. 2
Type UGS Watertight Underground Splice Kit consists of a standard AMS
splicer/reducer and two heavy wall heat-shrink sleeves.
Catalog Figure Conductor Range
Number Number Minimum Maximum
UGSKIT2* 1 8 AWG 2 AWG
UGSKIT250* 2 1 AWG 250 kcmil
* UL486D Listed for Direct Burial.
TYPE UGSKIT8
UF Direct Burial Splice Kit
For All Aluminum or
Copper/Aluminum Combinations
Type UGS UF Splice Kit consists of a UF splice connector and a heavy wall
heatshrink sleeve.
Catalog Number Conductor Range Copper
UGSKIT8* 14 - 8 AWG
* UL486D Listed for Direct Burial.
10 11
Mechanical BURNDY® EC&M BURNDY® EC&M Mechanical
U-BLOK™ POWER SPEC-BLOK™
DISTRIBUTION BLOCKS POWER DISTRIBUTION
For Junction Box Applications CONNECTORS
The U-BLOK™ system is a modern, state-of-the-art FT4B500 Unique, modular, made-to-order, power-distribution
approach to multi-load power distribution assemblies accommodate any number of supply
applications. Among typical uses are multi-story or and load conductors in any number of poles.
multi-unit buildings, HVAC, refrigeration, control Capacity matches the conductors accommodated
panels, motor control, switch gear, elevator systems and SPEC-BLOK™ assures uniform loading.
and materials-handling equipment. U-BLOK™ is UL
Adjacent poles are separated by easy-to-handle,
Listed for Copper or Aluminum conductors and rated FT3B4/0 wrap-around insulating covers which eliminate
for 600-volt applications. U-BLOK™ can be
taping and reduce heat build-up by allowing air to
mounted on bases for use in troughs or bolted
flow freely around connectors. SPEC-BLOK™ is
directly to junction boxes. AL9CU rated.
UL Listed for copper or aluminum conductors for
600 volts (AL9CU). Assemblies are mounted on
platforms suitable for easy installation in wireway
3S or junction box.
*
3S 3U
3 WIRE POWER DISTRIBUTION BLOCKS
Maximum Features and Benefits
• Accommodate unlimited conductors.
Number of Al or Cu Allen Tor-
〫 Fits wide range of applications.
Catalog Wires per Wire Range * Weight Wrench que Strip
• Connector elements tin-plated.
Number Phase Run Tap W L H Each Size (in-lb) Length 〫 Provides high reliability, low-resistance connections.
FT3B4/0 3/0 - 4/0 6 - 4/0 1-1/4 lbs. 1/4 200 1-1/2 • User friendly, space-saving design.
2 3-7/8 5-7/8 4-1/8
FT3B500 400 - 500 6 - 500 2-1/8 lbs. 3/8 375 2-5/16 〫 Easy to install. Saves labor.
3S 2 250-350 6 - 350 3-5/8 9-3/4 4-7/8 3 lbs. 1/4 200 1-3/4 • 94-VO rated insulation folds into place insulating the components.
Run Tap Run Tap 〫 Saves time and material. Allows easy installation.
3U 1 8 3/0-500 6 - 1/0 4-3/4 9-3/4 5-1/2 3 lbs. 5/32 110 2-5/161-5/32 • Connector caps removable for easy cable lay-in.
〫 Saves labor. Makes installation easier. Allows installation or straight-
4 WIRE POWER DISTRIBUTION BLOCKS through conductors. Eases retrofit.
Maximum • Belleville washers built-in on pressure screw assemblies, except in
assemblies installed with a 5/32 Allen wrench.
Number of Al or Cu Allen Tor-
〫 Provides high-integrity connections.
Catalog Wires per Wire Range * Weight Wrench que Strip
• Conductors can be cut or fed straight through.
Number Phase Run Tap W L H Each Size (in-lb) Length 〫 Straight through installation ideal for riser applications.
FT4B4/0 3/0 - 4/0 6 - 4/0 2 lbs. 1/4 200 1-1/2
2 3-7/8 7-7/8 4-1/8
FT4B500 400 - 500 6 - 500 2-3/4 lbs. 3/8 375 2-5/16 * SPEC-BLOK™ catalog assembly not UL Listed, individual SPEC-BLOK™ connectors are UL Listed.
4S 2 250-350 6 - 350 3-5/8 11-3/4 4-7/8 3-1/2 lbs. 1/4 200 1-3/4 See next page.
Run Tap Run Tap
4U 1 8 3/0-500 6 - 1/0 4-3/4 11-3/4 5-1/2 3-1/2 lbs. 5/32 110 2-5/161-5/32
PENETROX™ inhibitor is recommended for all aluminum wire connections. Contact BURNDY® Technical Services: 1-800-451-4956,
For two wire tap range is 8 through 1/0.
* Aluminum and copper conductors cannot be assembled under the same pressure plate or t-bar. or BURNDY® Customer Service: 1-800-346-4175
U-BLOK™ MOUNTING PLATFORMS
For Trough Applications
Catalog Gutter Weight
Number W L H Size Each
TBPT-6* 4-1/4 5-7/8 1-1/2 6 3/4 lb.
TBPT-8 4-1/4 7-7/8 3-1/2 8 1-1/4 lb.
TBPT-10 4-1/4 9-7/8 4 10 1-1/2 lb.
TBPT-12 4-1/4 11-7/8 4 12 1-3/4 lb.
Hole pattern shown is for reference only.
* Supercedes TBPT4/0-6 and TBPT350/500-6.
12 13
Mechanical BURNDY® EC&M BURNDY® EC&M Mechanical
SPEC-BLOK™ POWER SPEC-BLOK™
DISTRIBUTION CONNECTORS Mounting Platforms
* SPEC-BLOK™ Mounting Platforms are rigid steel construction with a black
finish. They can be supplied for junction box mounting or wireway construc-
tion allowing trough conductors to pass underneath the assemblies.
Features and Benefits
• The SPEC-BLOK™ system includes 12 connector elements
What’s New in the MaxBulk Mailer 5.72 serial key or number?
Screen Shot
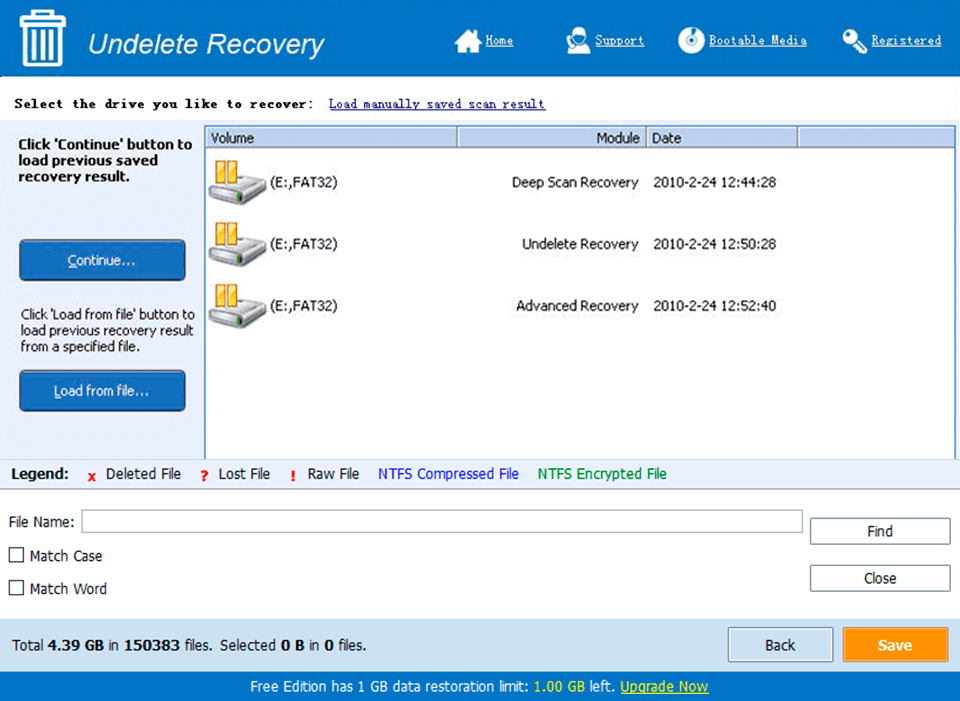
System Requirements for MaxBulk Mailer 5.72 serial key or number
- First, download the MaxBulk Mailer 5.72 serial key or number
-
You can download its setup from given links: